Geology And Geophysics Research Team Wins GCAGS And GCSSEPM Grover E. Murray Award
Their research focuses on TOC-rich zones in the Eagle Ford Shale.
Nov 15, 2021
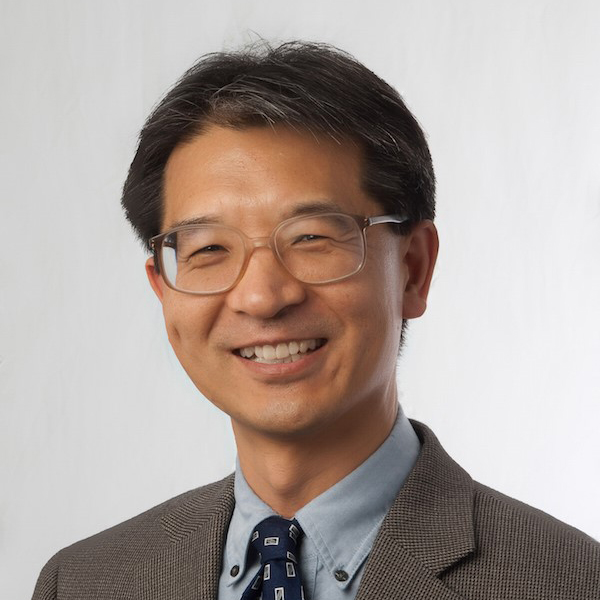
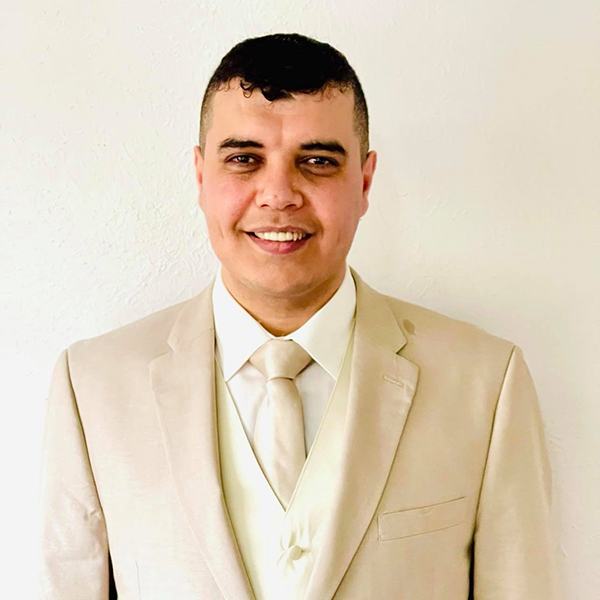
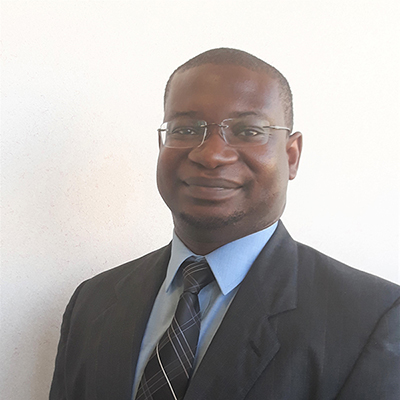
A team of researchers from the Department of Geology and Geophysics, including graduate students Adewale Amosu and Mohamed Imsalem, and Dr. Yuefeng Sun, professor, were recently recognized for their paper, “Machine Learning Identification of TOC–Rich Zones in the Eagle Ford Shale” at the 70th Gulf Coast Association of Geological Societies (GCAGS) and Gulf Coast Section of Society of Sedimentary Geology (GCSSEPM) Convention and Exposition - GEOGULF 2020, held Sept. 30 – Oct. 2, in Lafayette, Louisiana. They received the 3rd Place Grover E. Murray award for Best Published Paper.
Production from tight oil and shale gas plays has transformed the energy industry in the United States, the researchers said. The viability of tight oil plays relies heavily on identifying the most suitable geologic formation with optimal petrophysical and mechanical properties.
This project addresses the crucial problem of identifying productive source rocks, characterized by high organic content and brittle zones, in unconventional resource plays, using machine learning. Machine learning algorithms analyze large amounts of data to derive patterns, statistical rules, and functional mappings, which are then applied to complete specific tasks.
It is projected that machine learning and artificial intelligence techniques will significantly transform many aspects of the energy industry, the team said. Apart from taking over repetitive tasks, the application of artificial intelligence techniques will lead to new insights to improve efficiency in every aspect of exploration, production, and distribution, they said.
The study uses a newly developed robust data-driven support vector machine learning workflow that relies on core measured data and petrophysical data to predict total organic carbon (TOC) and thermal maturity at depth intervals where geochemical data is missing. Core measured data are more reliable than data from other sources and are considered the ground truth.
The method successfully identifies thermally mature TOC-rich zones and matches well with other methods and geological observations. Further tests combine data from multiple shale oil plays. The machine learning methodology again successfully classified the test data set with predicted TOC-rich zones matching the independently obtained measured core TOC measurements. This is quite significant as it demonstrates the cross-basin applicability of the method. The method can also be used to predict other geochemical or geomechanical properties of interest.
The team plans to extend machine learning applications to seismic data, integrating it with a rock physics-based seismic inversion workflow for applications such as carbon capture and storage and enhanced oil recovery. Pre-stack and post-stack seismic inversion are routinely used to estimate acoustic and elastic impedance from seismic data. The Sun rock physics model, developed by Professor Yuefeng Sun, extends the Biot theory of poroelasticity and defines a key effective pore structure parameter called the frame flexibility factor (illustrated below), which can be used to quantify the variation of pore structure on core and log scales and invert carbonate reservoir pore structure and permeability from seismic data. The figure below illustrates the effect of pore-structure on velocity and impedance, captured by the frame flexibility factor.
By John Sarao
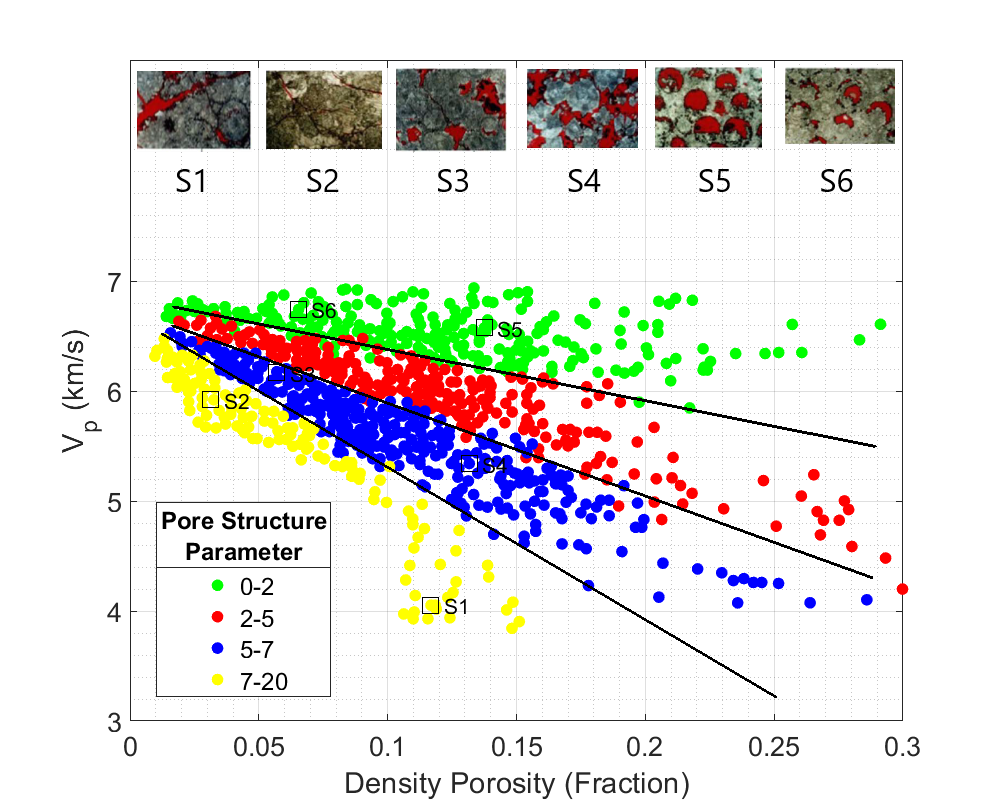